No-Nonsense Talk about AI in ERP
- Charles Rathmann
- Jul 22, 2024
- 2 min read
Updated: Jul 23, 2024
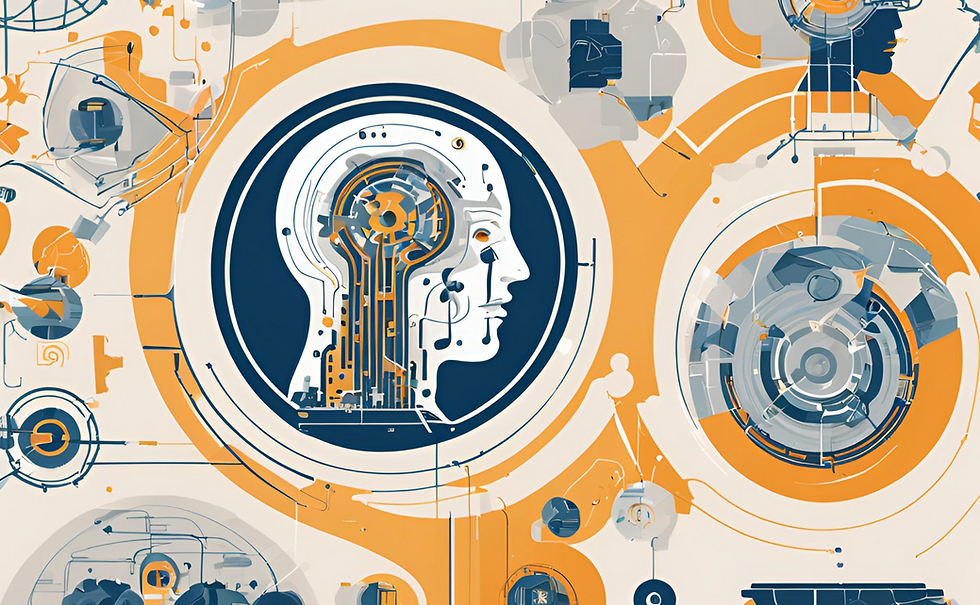
Let's be clear. Artificial intelligence (AI) has been creeping into enterprise resource planning (ERP) software for a decade. Early on, something as simple as automatic inventory re-order points may have been described as AI by a vendor.
Same thing for invoice matching in accounts payable. We start to see more dynamic approaches to AI once we look out to the four walls of the business, with scheduling options for field service and construction coming to market either within the enterprise suite or as integrated tools. More advanced algorithms may constitute a more formal type of AI in ERP, and machine learning certainly qualifies.
But What About Generative AI?
Writes Tony Bradley on Forbes: "Generative AI is poised to disrupt the ERP market, promising a future where ERP systems are not just tools for managing business operations but catalysts for growth, innovation, and strategic advantage. The future of enterprise resource planning will be redefined by more efficient, adaptable, and accessible solutions."
Forrester VP and Principal Analyst Liz Herbert writes in a recent blog post: GenAI will free finance and operations employees from cumbersome tasks such as narrative reporting, customer collection emails, and account summarization. Instead of monotonously and manually performing these tasks themselves, employees will act as human reviewers of the AI-generated work. This will afford employees more free time to dedicate to other tasks, increasing overall productivity."
Herbert wisely suggests the generative AI will be a guide-on-the-side, offering options for humans to review, probably taking some time to train the application how to create valid material. But with the mission-critical nature of work that takes place in ERP, generative AI will need to become auditable so results can be explained.
As Saurabh Bagchi, a professor of electrical and computer engineering at Purdue University explains in Scientific American, this creates challenges in a business application.
"Any of the three components of a machine-learning system can be hidden, or in a black box," Bagchi writes. "As is often the case, the algorithm is publicly known, which makes putting it in a black box less effective. So to protect their intellectual property, AI developers often put the model in a black box. Another approach software developers take is to obscure the data used to train the model – in other words, put the training data in a black box."
Explainable ML
Algorithms that improve with time, machine learning, are also valuable, but cataloging what the machine learning engine in fact learns and how it applies it to business problems should still be explainable.
Apart from ensuring that the algorithms and training data an AI relies on are in what is called a "glass box," buyers interested in AI in ERP may be well-served to consider more established types of AI as the most elegant solution.
Old School AI
Generative AI is cool, but more established technology may prove to be a better fit for most ERP-oriented applications. Configurable rules engines give software users the ability to define under what conditions certain actions become desirable. These engines also will enable users to create multiple rules and set priorities for how the AI makes decisions or recommendations. This will be explainable, configurable, and eliminate the "black box" problem.
Comments